5 releases
new 0.7.1 | Sep 13, 2024 |
---|---|
0.6.15 | Sep 12, 2024 |
0.6.11 | Aug 20, 2024 |
0.6.10 | Aug 20, 2024 |
0.6.9 | Aug 20, 2024 |
#32 in Biology
602 downloads per month
1MB
4K
SLoC
Kun-peng 
Here, we introduce Kun-peng, an ultra-memory-efficient metagenomic classification tool (Fig. 1). Inspired by Kraken2's k-mer-based approach, Kun-peng employs algorithms for minimizer generation, hash table querying, and classification. The cornerstone of Kun-peng's memory efficiency lies in its unique ordered block design for reference database. This strategy dramatically reduces memory usage without compromising speed, enabling Kun-peng to be executed on both personal computers and HPCP for most databases. Moreover, Kun-peng incorporates an advanced sliding window algorithm for sequence classifications to reduce the false-positive rates. Finally, Kun-peng supports parallel processing algorithms to further bolster its speed. Kun-peng offers two classification modes: Memory-Efficient Mode (Kun-peng-M) and Full-Speed Mode (Kun-peng-F). Remarkably, Kun-peng-M achieves a comparable processing time to Kraken2 while using less than 10% of its memory. Kun-peng-F loads all the database blocks simultaneously, matching Kraken2's memory usage while surpassing its speed. Notably, Kun-peng is compatible with the reference database built by Kraken2 and the associated abundance estimate tool Bracken1, making the transition from Kraken2 effortless. The name "Kun-peng" was derived from Chinese mythology and refers to a creature transforming between a giant fish (Kun) and a giant bird (Peng), reflecting the software's flexibility in navigating complex metagenomic data landscapes.
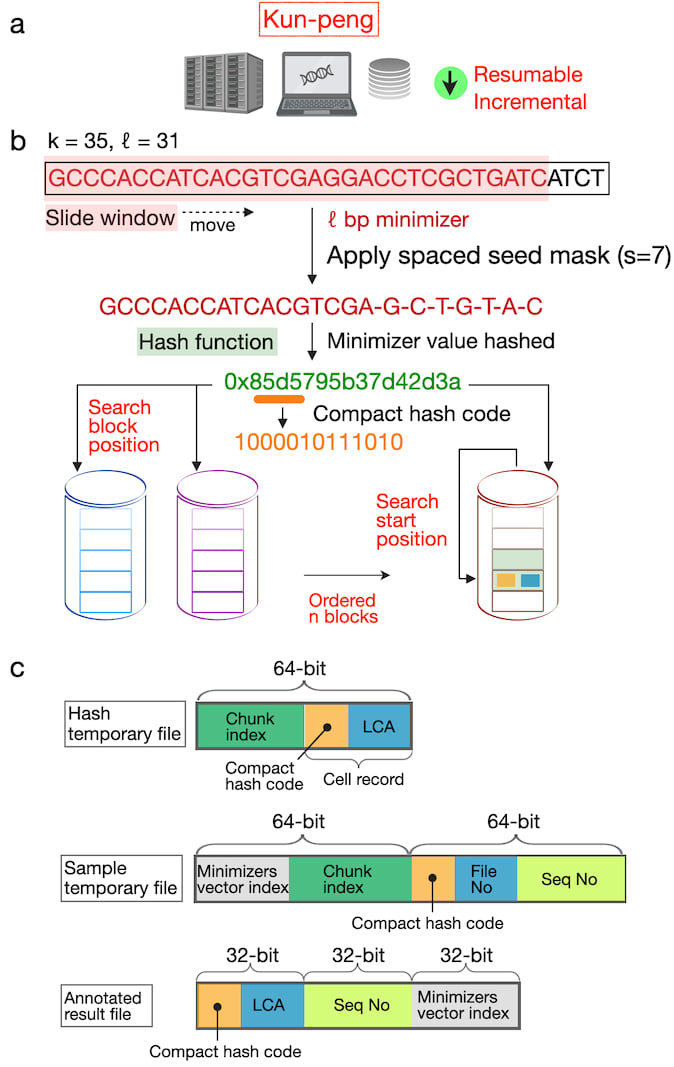
Fig. 1. Overview of the algorithms of Kun-peng.
To assess Kun-peng's accuracy and performance, we used two datasets comprising 14 mock metagenomes 2,3. We processed these data with Kraken2 4 and Centrifuge 5, both requiring relatively lower memory and supporting custom databases. The classification processes were executed with default parameters, generating reports of identified taxa and their abundance estimated by Bracken 1. As most classifiers considered below 0.01% abundance false positives, we removed these taxa for simplicity 6.
Critical metrics for metagenomic classification include precision, recall, and the area under the precision-recall curve (AUPRC). After filtering the low-abundance species, these metrics were calculated at the genus or species level. All tools performed better at the genus level, with performance decreasing at the species level (Fig. 2a). At the genus level, Centrifuge's precision was 25.5 ± 12.4 % lower than Kun-peng's (Fig. 2a). At the species level, Kun-peng significantly outperformed Kraken2 and Centrifuge, showing 11.2 ± 8.08 % and 23.6 ± 12.3 % higher precision, respectively (Fig. 2a). We focused on the genus level due to higher overall performance. Kun-peng's increased precision resulted from significantly lower false positives compared to Kraken2 and Centrifuge, which showed 16.9 ± 10.0 % and 61.7 ± 40.2 % higher false positives, respectively (Fig. 2b).
We constructed a standard database using the complete RefSeq genomes of archaeal, bacterial, and viral domains. The database contains 123GB of fasta sequences, generating a 56GB hash index for Kraken2 and Kun-peng and a 72GB database index for Centrifuge. Database construction time for Kun-peng was noticeably longer due to sub-hashing calculations (Fig. 2c). However, Kun-peng required only 4.6GB of peak memory, roughly 8.19% and 1.50% of Kraken2 and Centrifuge's peak memory, for database construction (Fig. 2c).
Kun-peng offers two modes for taxonomy classification: Memory-Efficient Mode (Kun-peng-M) and Full-Speed Mode (Kun-peng-F), with identical classification results. Kun-peng-M matches Kraken2's processing time and uses 57.0 ± 2.25 % of Centrifuge's time (Fig. 2d). However, Kun-peng-M requires only 4.5 ± 1.1 GB peak memory, which is 7.96 ± 0.19 % and 6.31 ± 0.15 % of Kraken2 and Centrifuge's peak memory, respectively (Fig. 2d). Compared to Kraken2, the Kun-peng-F consumes the same memory but requires only of the 67.2 ± 4.57 % processing time. Compared to Centrifuge, Kun-peng-F uses 77.9 ± 0.22 % memory while requiring only 38.8 ± 4.25 % of its processing time (Fig. 2d). Remarkably, with an ultra-low memory requirement, Kun-peng-M can even operate on most personal computers when the standard reference database is used (Fig. 2e).
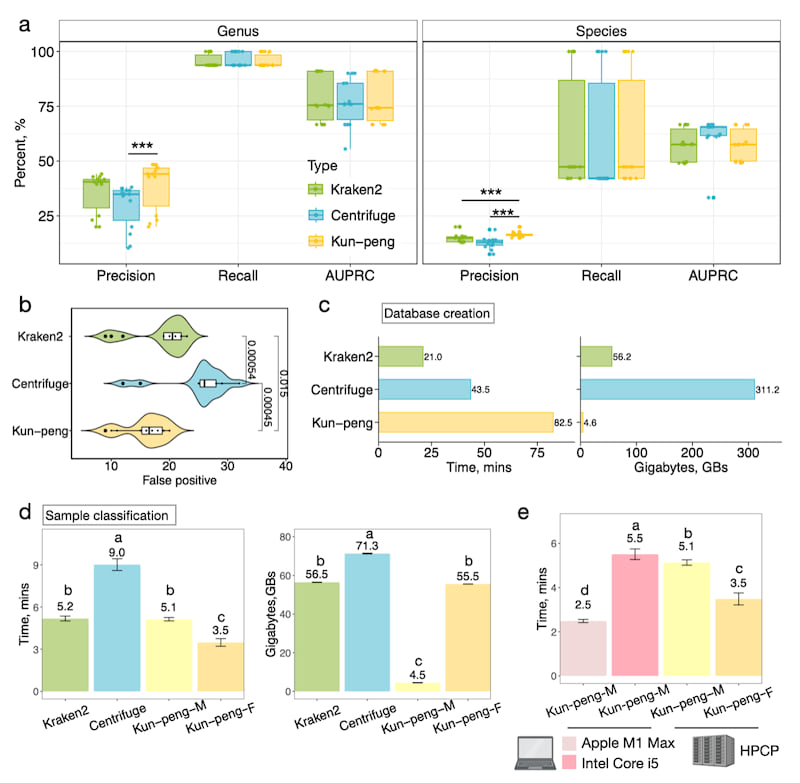
Fig. 2. Performance benchmark of Kun-peng against other metagenomic classifiers.
References:
- Lu, J., Breitwieser, F. P., Thielen, P. & Salzberg, S. L. Bracken: Estimating species abundance in metagenomics data. PeerJ Comput. Sci. 2017, 1–17 (2017).
- Amos, G. C. A. et al. Developing standards for the microbiome field. Microbiome 8, 1–13 (2020).
- Kralj, J., Vallone, P., Kralj, J., Hunter, M. & Jackson, S. Reference Material 8376 Microbial Pathogen DNA Standards for Detection and Identification NIST Special Publication 260-225 Reference Material 8376 Microbial Pathogen DNA Standards for Detection and Identification.
- Wood, D. E., Lu, J. & Langmead, B. Improved metagenomic analysis with Kraken 2. Genome Biol. 20, (2019).
- Kim, D., Song, L., Breitwieser, F. P. & Salzberg, S. L. Centrifuge: Rapid and sensitive classification of metagenomic sequences. Genome Res. 26, 1721–1729 (2016).
- Ye, S. H., Siddle, K. J., Park, D. J. & Sabeti, P. C. Benchmarking Metagenomics Tools for Taxonomic Classification. Cell 178, 779–794 (2019).
Get Started
Follow these steps to install Kun-peng and run the examples.
Method 1: Download Pre-built Binaries (Recommended)
If you prefer not to build from source, you can download the pre-built binaries for your platform from the GitHub releases page.
For Linux users (CentOS 7 compatible):
# Replace X.Y.Z with the latest version number
VERSION=vX.Y.Z
mkdir kun_peng_$VERSION
wget https://github.com/eric9n/Kun-peng/releases/download/$VERSION/kun_peng-$VERSION-centos7
# For linux x86_64
# wget https://github.com/eric9n/Kun-peng/releases/download/$VERSION/kun_peng-$VERSION-x86_64-unknown-linux-gnu
mv kun_peng-$VERSION-centos7 kun_peng_$VERSION/kun_peng
chmod +x kun_peng_$VERSION/kun_peng
# Add to PATH
echo "export PATH=\$PATH:$PWD/kun_peng_$VERSION" >> ~/.bashrc
source ~/.bashrc
For macOS users:
Homebrew
brew install eric9n/tap/kun_peng
Donwload binary
# Replace X.Y.Z with the latest version number
VERSION=vX.Y.Z
mkdir kun_peng_$VERSION
# For Intel Macs
wget https://github.com/eric9n/Kun-peng/releases/download/$VERSION/kun_peng-$VERSION-x86_64-apple-darwin
mv kun_peng-$VERSION-x86_64-apple-darwin kun_peng_$VERSION/kun_peng
# For Apple Silicon Macs
# wget https://github.com/eric9n/Kun-peng/releases/download/$VERSION/kun_peng-$VERSION-aarch64-apple-darwin
# mv kun_peng-$VERSION-aarch64-apple-darwin kun_peng_$VERSION/kun_peng
chmod +x kun_peng_$VERSION/kun_peng
# Add to PATH
echo "export PATH=\$PATH:$PWD/kun_peng_$VERSION" >> ~/.zshrc # or ~/.bash_profile for Bash
source ~/.zshrc # or source ~/.bash_profile for Bash
For Windows users:
# Replace X.Y.Z with the latest version number
$VERSION = "vX.Y.Z"
New-Item -ItemType Directory -Force -Path kun_peng_$VERSION
Invoke-WebRequest -Uri "https://github.com/eric9n/Kun-peng/releases/download/$VERSION/kun_peng-$VERSION-x86_64-pc-windows-msvc.exe" -OutFile "kun_peng_$VERSION\kun_peng.exe"
# Add to PATH
$env:Path += ";$PWD\kun_peng_$VERSION"
[Environment]::SetEnvironmentVariable("Path", $env:Path, [EnvironmentVariableTarget]::User)
After installation, you can verify the installation by running:
kun_peng --version
Run the kun_peng
example
We will use a very small virus database on the GitHub homepage as an example:
- clone the repository
git clone https://github.com/eric9n/Kun-peng.git
cd Kun-peng
- build database
kun_peng build --download-dir data/ --db test_database
merge fna start...
merge fna took: 29.998258ms
estimate start...
estimate count: 14080, required capacity: 31818.0, Estimated hash table requirement: 124.29KB
convert fna file "test_database/library.fna"
process chunk file 1/1: duration: 29.326627ms
build k2 db took: 30.847894ms
- classify
# temp_chunk is used to store intermediate files
mkdir temp_chunk
# test_out is used to store output files
mkdir test_out
kun_peng classify --db test_database --chunk-dir temp_chunk --output-dir test_out data/COVID_19.fa
hash_config HashConfig { value_mask: 31, value_bits: 5, capacity: 31818, size: 13051, hash_capacity: 1073741824 }
splitr start...
splitr took: 18.212452ms
annotate start...
chunk_file "temp_chunk/sample_1.k2"
load table took: 548.911µs
annotate took: 12.006329ms
resolve start...
resolve took: 39.571515ms
Classify took: 92.519365ms
Method 2: Clone the Repository and Build the project
Prerequisites
- Rust: This project requires the Rust programming environment if you plan to build from source.
Build the Projects
First, clone this repository to your local machine:
git clone https://github.com/eric9n/Kun-peng.git
cd kun_peng
Ensure that both projects are built. You can do this by running the following command from the root of the workspace:
cargo build --release
This will build the kr2r and ncbi project in release mode.
Run the kun_peng
example
Next, run the example script that demonstrates how to use the kun_peng
binary. Execute the following command from the root of the workspace:
cargo run --release --example build_and_classify
This will run the build_and_classify.rs example located in the kr2r project's examples directory.
Example Output You should see output similar to the following:
Executing command: /path/to/workspace/target/release/kun_peng build --download-dir data/ --db test_database
kun_peng build output: [build output here]
kun_peng build error: [any build errors here]
Executing command: /path/to/workspace/target/release/kun_peng direct --db test_database data/COVID_19.fa
kun_peng direct output: [direct output here]
kun_peng direct error: [any direct errors here]
This output confirms that the kun_peng
commands were executed successfully and the files were processed as expected.
ncbi_dl tool
For detailed information and usage instructions for the ncbi_dl tool, please refer to the ncbi_dl repository.
The ncbi_dl tool is used to download resources from the NCBI website, including taxonomy files and genome data. It provides a convenient way to obtain the necessary data for building Kun-peng databases.
Downloading Genome Databases
To download genome databases using ncbi_dl, you can use the genomes
(or gen
) command. Here's a basic example:
ncbi_dl -d /path/to/download/directory gen -g bacteria
This command will download bacterial genomes to the specified directory. You can replace bacteria
with other genome groups like archaea
, fungi
, protozoa
, or viral
depending on your needs.
Some key options for the genomes
command include:
-g, --groups <GROUPS>
: Specify which genome groups to download (e.g., bacteria, archaea, viral)-f, --file-types <FILE_TYPES>
: Choose which file types to download (default is genomic.fna.gz)-l, --assembly-level <ASSEMBLY_LEVEL>
: Set the assembly level (e.g., complete, chromosome, scaffold, contig)
For a full list of options and more detailed usage instructions, please refer to the ncbi_dl repository documentation.
For installation, additional usage examples, and more detailed documentation, please visit the ncbi_dl repository linked above.
kun_peng
Usage: kun_peng <COMMAND>
Commands:
estimate estimate capacity
build build `k2d` files
hashshard Convert Kraken2 database files to Kun-peng database format for efficient processing and analysis.
splitr Split fast(q/a) file into ranges
annotate annotate a set of sequences
resolve resolve taxonomy tree
classify Integrates 'splitr', 'annotate', and 'resolve' into a unified workflow for sequence classification. classify a set of sequences
direct Directly load all hash tables for classification annotation
merge-fna A tool for processing genomic files
help Print this message or the help of the given subcommand(s)
Options:
-h, --help Print help
-V, --version Print version
build database
Build the kun_peng database like Kraken2, specifying the directory for the data files downloaded from NCBI, as well as the database directory.
./target/release/kun_peng build -h
build database
Usage: kun_peng build [OPTIONS] --download-dir <DOWNLOAD_DIR> --db <DATABASE>
Options:
-d, --download-dir <DOWNLOAD_DIR>
Directory to store downloaded files
--db <DATABASE>
ncbi library fna database directory
-k, --k-mer <K_MER>
Set length of k-mers, k must be positive integer, k=35, k cannot be less than l [default: 35]
-l, --l-mer <L_MER>
Set length of minimizers, 1 <= l <= 31 [default: 31]
--minimizer-spaces <MINIMIZER_SPACES>
Number of characters in minimizer that are ignored in comparisons [default: 7]
-T, --toggle-mask <TOGGLE_MASK>
Minimizer ordering toggle mask [default: 16392584516609989165]
--min-clear-hash-value <MIN_CLEAR_HASH_VALUE>
-r, --requested-bits-for-taxid <REQUESTED_BITS_FOR_TAXID>
Bit storage requested for taxid 0 <= r < 31 [default: 0]
-p, --threads <THREADS>
Number of threads [default: 10]
--cache
estimate capacity from cache if exists
--max-n <MAX_N>
Set maximum qualifying hash code [default: 4]
--load-factor <LOAD_FACTOR>
Proportion of the hash table to be populated (build task only; def: 0.7, must be between 0 and 1) [default: 0.7]
-h, --help
Print help
-V, --version
Print version
Convert Kraken2 database
This tool converts Kraken2 database files into Kun-peng database format for more efficient processing and analysis. By specifying the database directory and the hash file capacity, users can control the size of the resulting database index files.
./target/release/kun_peng hashshard -h
Convert Kraken2 database files to Kun-peng database format for efficient processing and analysis.
Usage: kun_peng hashshard [OPTIONS] --db <DATABASE>
Options:
--db <DATABASE> The database directory for the Kraken 2 index. contains index files(hash.k2d opts.k2d taxo.k2d)
--hash-capacity <HASH_CAPACITY> Specifies the hash file capacity.
Acceptable formats include numeric values followed by 'K', 'M', or 'G' (e.g., '1.5G', '250M', '1024K').
Note: The specified capacity affects the index size, with a factor of 4 applied.
For example, specifying '1G' results in an index size of '4G'.
Default: 1G (capacity 1G = file size 4G) [default: 1G]
-h, --help Print help
-V, --version Print version
classify
The classification process is divided into three modes:
- Direct Processing Mode:
- Description: In this mode, all database files are loaded simultaneously, which requires a significant amount of memory. Before running this mode, you need to check the total size of hash_*.k2d files in the database directory using the provided script. Ensure that your available memory meets or exceeds this size.
bash cal_memory.sh $database_dir
- Characteristics:
- High memory requirements
- Fast performance
Command Help
./target/release/kun_peng direct -h
Directly load all hash tables for classification annotation
Usage: kun_peng direct [OPTIONS] --db <DATABASE> [INPUT_FILES]...
Arguments:
[INPUT_FILES]... A list of input file paths (FASTA/FASTQ) to be processed by the classify program. Supports fasta or fastq format files (e.g., .fasta, .fastq) and gzip compressed files (e.g., .fasta.gz, .fastq.gz)
Options:
--db <DATABASE>
database hash chunk directory and other files
-P, --paired-end-processing
Enable paired-end processing
-S, --single-file-pairs
Process pairs with mates in the same file
-Q, --minimum-quality-score <MINIMUM_QUALITY_SCORE>
Minimum quality score for FASTQ data [default: 0]
-T, --confidence-threshold <CONFIDENCE_THRESHOLD>
Confidence score threshold [default: 0]
-K, --report-kmer-data
In comb. w/ -R, provide minimizer information in report
-z, --report-zero-counts
In comb. w/ -R, report taxa w/ 0 count
-g, --minimum-hit-groups <MINIMUM_HIT_GROUPS>
The minimum number of hit groups needed for a call [default: 2]
-p, --num-threads <NUM_THREADS>
The number of threads to use [default: 10]
--output-dir <KRAKEN_OUTPUT_DIR>
File path for outputting normal Kraken output
-h, --help
Print help (see more with '--help')
-V, --version
Print version
- Chunk Processing Mode:
- Description: This mode processes the sample data in chunks, loading only a small portion of the database files at a time. This reduces the memory requirements, needing a minimum of 4GB of memory plus the size of one pair of sample files.
- Characteristics:
- Low memory consumption
- Slower performance compared to Direct Processing Mode
Command Help
./target/release/kun_peng classify -h
Integrates 'splitr', 'annotate', and 'resolve' into a unified workflow for sequence classification. classify a set of sequences
Usage: kun_peng classify [OPTIONS] --db <DATABASE> --chunk-dir <CHUNK_DIR> [INPUT_FILES]...
Arguments:
[INPUT_FILES]... A list of input file paths (FASTA/FASTQ) to be processed by the classify program. Supports fasta or fastq format files (e.g., .fasta, .fastq) and gzip compressed files (e.g., .fasta.gz, .fastq.gz)
Options:
--db <DATABASE>
--chunk-dir <CHUNK_DIR>
chunk directory
--output-dir <KRAKEN_OUTPUT_DIR>
File path for outputting normal Kraken output
-P, --paired-end-processing
Enable paired-end processing
-S, --single-file-pairs
Process pairs with mates in the same file
-Q, --minimum-quality-score <MINIMUM_QUALITY_SCORE>
Minimum quality score for FASTQ data [default: 0]
-p, --num-threads <NUM_THREADS>
The number of threads to use [default: 10]
--buffer-size <BUFFER_SIZE>
[default: 16777216]
--batch-size <BATCH_SIZE>
The size of each batch for processing taxid match results, used to control memory usage
[default: 16]
-T, --confidence-threshold <CONFIDENCE_THRESHOLD>
Confidence score threshold [default: 0]
-g, --minimum-hit-groups <MINIMUM_HIT_GROUPS>
The minimum number of hit groups needed for a call [default: 2]
--kraken-db-type
Enables use of a Kraken 2 compatible shared database
-K, --report-kmer-data
In comb. w/ -R, provide minimizer information in report
-z, --report-zero-counts
In comb. w/ -R, report taxa w/ 0 count
-h, --help
Print help (see more with '--help')
-V, --version
Print version
- Step-by-Step Processing Mode:
- Description: This mode breaks down the chunk processing mode into individual steps, providing greater flexibility in managing the entire classification process.
- Characteristics:
- Flexible processing steps
- Similar memory consumption to Chunk Processing Mode
- Performance varies based on execution steps
Output
- test_out/output_1.txt:
Standard Kraken Output Format:
- "C"/"U": a one letter code indicating that the sequence was either classified or unclassified.
- The sequence ID, obtained from the FASTA/FASTQ header.
- The taxonomy ID Kraken 2 used to label the sequence; this is 0 if the sequence is unclassified.
- The length of the sequence in bp. In the case of paired read data, this will be a string containing the lengths of the two sequences in bp, separated by a pipe character, e.g. "98|94".
- A space-delimited list indicating the LCA mapping of each k-mer in the sequence(s). For example, "562:13 561:4 A:31 0:1 562:3" would indicate that:
- the first 13 k-mers mapped to taxonomy ID #562
- the next 4 k-mers mapped to taxonomy ID #561
- the next 31 k-mers contained an ambiguous nucleotide
- the next k-mer was not in the database
- the last 3 k-mers mapped to taxonomy ID #562
Note that paired read data will contain a "
|:|
" token in this list to indicate the end of one read and the beginning of another.
- test_out/output_1.kreport2:
100.00 1 0 R 1 root
100.00 1 0 D 10239 Viruses
100.00 1 0 D1 2559587 Riboviria
100.00 1 0 O 76804 Nidovirales
100.00 1 0 O1 2499399 Cornidovirineae
100.00 1 0 F 11118 Coronaviridae
100.00 1 0 F1 2501931 Orthocoronavirinae
100.00 1 0 G 694002 Betacoronavirus
100.00 1 0 G1 2509511 Sarbecovirus
100.00 1 0 S 694009 Severe acute respiratory syndrome-related coronavirus
100.00 1 1 S1 2697049 Severe acute respiratory syndrome coronavirus 2
Sample Report Output Formats:
- Percentage of fragments covered by the clade rooted at this taxon
- Number of fragments covered by the clade rooted at this taxon
- Number of fragments assigned directly to this taxon
- A rank code, indicating (U)nclassified, (R)oot, (D)omain, (K)ingdom, (P)hylum, (C)lass, (O)rder, (F)amily, (G)enus, or (S)pecies. Taxa that are not at any of these 10 ranks have a rank code that is formed by using the rank code of the closest ancestor rank with a number indicating the distance from that rank. E.g., "G2" is a rank code indicating a taxon is between genus and species and the grandparent taxon is at the genus rank.
- NCBI taxonomic ID number
- Indented scientific name
Dependencies
~7–16MB
~206K SLoC